%%
tags:: #state/boat #ProductGrowth #experimentation
started_date:: [[2023-03-12]]
published::
project:: #ProjectKnowledgeBase
up::
##### Research and idea capturing:
-
%%
[[Product Growth]] | Updated [[2023-03-12]]
# How many tests can we run?
###### Calculating Sample Size in three different ways.
> [!question] What's this about?
>
> This is an aggregate of all I have on the knowledge base for **sample size calculation** and **test capacity estimation**. This post will evolve as I learn and receive feedback.
## **We can run up to two tests per month. One sitewide with confidence and another if we group this page template.**
This was my answer to the Product Manager’s question.
**There are a couple of ways to reach the answer. I will show you three different.**
#### **—**
This question first came to me while navigating through the agency ranks at Ladder.io. Our CEO wanted a test capacity estimation in preparation for a client call.
At the time, I had very little intuition to answer and the statistical procedures were blurry. I didn’t know _[yet](https://www.youtube.com/watch?v=_X0mgOOSpLU&ab_channel=TED)._ Now I do, and it’s simple, but it can get complex.
Considering you have the right inputs, in 10 minutes or so you’ll have your answer.
If not, you’ll know the steps to get there and what groundwork to cover.
![[Pasted image 20230312175355.png]]
Hubbard’s reference to Barry Nussbaum chief statistician at the Environmental Protection Agency (EPA).
## Who's this post for? Will I get value from this?
Data-savvy experimenters and marketeers looking to answer a specific question for their experimentation programs.
I received positive feedback from data analysts and conversion specialists.
Don't like the numbers discussion? I understand.
Please share with someone in your team that would get value from it and hopefully help you clarify test design and analysis 🚀
**Share this post.**
## The 6 questions answered:
For exercises like this, I like to look back and codify all the questions I was able to answer throughout the process so it becomes a playbook.
Here are the ones I took note of:
- When do we need to know test capacity?
- What do we need to start estimating sample size?
- First principles, what are we trying to do exactly?
- Percentage or continuous metrics?
- Online calculators, spreadsheets, or formulas?
- Test capacity, why does it matter?
![[Pasted image 20230312175455.png]]
### When do we need to know the test capacity?
> **Test capacity** is the number of tests the product unit could run per month.
From my experience, “How many tests can I run?” appeared mostly for auditing purposes on the agency side and during ideation and prioritization on the product side.
![[Pasted image 20230312175538.png]]
[Reforge](https://www.reforge.com/experimentation-testing) process for scalable strategic experimentation.
So we’re either:
1. Supporting a Product Leader about how viable a test they have in mind is in terms of time duration;
2. Estimating how many experiments a product could run per month;
3. Designing an experiment to set in the agenda when to check test results;
4. Understanding experiment viability when considering traffic vs. MDE;
5. Thinking about prioritizing one test over another.
### **A brief discussion about experimentation stages**
> **Experimentation stages** the different steps that a team must go through from idea to decision.
![[Pasted image 20230312175611.png]]
Experimentation at its core is the application of the scientific method to answer relevant business questions.
Relevant in this scope means to reach a decision, resolution after consideration, that carries inherent risk, e.g., financial or public relations damage.
![[Pasted image 20230312175651.png]]
The scientific method starts with a relevant question
#### The process in eight steps:
1. Define a question;
2. Gather information, resources, and evidence (observe);
3. Form an explanatory hypothesis for a potential change;
4. Test the hypothesis by performing an experiment and collecting data;
5. Analyze the data;
6. Interpret the data and draw conclusions that serve as a starting point for a new hypothesis;
7. Communicate results;
8. Retest for reproducibility (frequently done by other scientists).
> Under this frame, sample size is calculated between steps 3 and 4.
![[Pasted image 20230312175738.png]]
### What do we need to start estimating sample size?
![[Pasted image 20230312175754.png]]
> [!attention] Beware
>
> The reasoning and calculations below assume a frequentist design to test analysis.
> More on this in the Bayesian vs. Frequentist section at the end.
First principles, what are we trying to do exactly?
1. We want to understand **how many samples are needed**,
2. so we know **how long to keep an experiment running**,
3. so we’re confident in our **ability to detect a pre-set minimum effect between control and variant with a certain confidence**.
#### Proportion or continuous metrics?
The sample size is calculated differently if your metric is a proportion (e.g., conversion rate %) of if it’s a continuous metric (e.g., ARPU or AOV).
![[Pasted image 20230312180020.png]]
### What are the components of test capacity?
#### General inputs
1. Overall evaluation criterion (OEC) and its baseline
2. Minimum Detectable Effect (MDE)
3. Confidence level (α) and Statistical Power (β)
4. Number of variants to be tested (N)
Percentage base metrics inputs
5. Weekly Active “Net” Users (WAnU)
Continuous metrics inputs
6. Baseline standard deviation (σ)
#### 1. Overall evaluation criterion (OEC)
![[Pasted image 20230312180217.png]]
Defining your primary metric is the starting point for sample size calculation.
OEC is the set of metrics and decision criteria used to judge the outcomes of A/B tests – which is predictive of long-term business and customer value and sensitive to the changes being tested.
Some OEC examples in places I worked:
- conversion to sign-up;
- conversion to product purchase;
- conversion to partner link click;
- conversion to wallet funded;
##### OEC Baseline
Now that you clarified your OEC, you must reach a baseline that you will use for the next calculations.
There are two possible scenarios:
1. Set it equal to the historical metric performance
2. Estimate based on projections
For example, if using option 1 we would set the baseline for this OEC at 8.17%
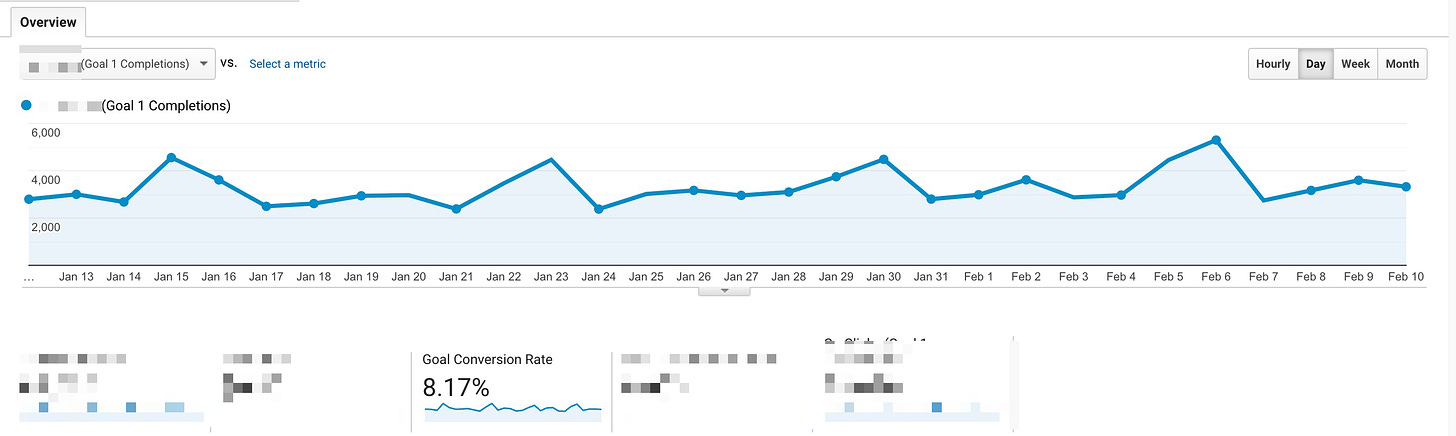
##### When to estimate on projections?
When there's no historical data available you will estimate the baseline metric based on projections.
It's not optimal, not exact, but it's the best you have to play with. **Your sample size will be an estimate.**
> **Trigger question: For the experience you want to optimize, what’s the best metric to measure in the short term that correlates to business success in the long term?**
_Full definition from [Analytics Toolkit](https://www.analytics-toolkit.com/) 👉🏼 **[What does "Overall Evaluation Criterion" mean?](https://www.analytics-toolkit.com/glossary/overall-evaluation-criterion/)**_
#### 2. Minimum Detectable Effect (MDE)
**MDE is the smallest improvement you are willing to be able to detect**. It determines how "sensitive" an experiment is.
> 👍🏼 Rule of thumb: The lower the baseline for your OEC the more samples you will need to get statistical significance.
I’ve worked with some teams that had some boundaries around determining MDEs to determine how “difficult” the experiment is:
- 1 % is considered simple
- 5 % medium
- >= 7% hard
I like to think about this using [[Experimentation portfolio spectrum|Reforge experimentation spectrum]] approach to a testing backlog. If the test is a big bet, you expect a big change in the MDE.
![[Pasted image 20230211170224.png]]
**Trigger question: How big of a difference actually matters to us from a business perspective? In other words, what change of the OEC is practically significant?**
_Full definition from [Analytics Toolkit](https://www.analytics-toolkit.com/) 👉🏼 **[What does "Minimum Detectable Effect" mean?](https://www.analytics-toolkit.com/glossary/minimum-detectable-effect/)**_
#### 3. Confidence level (α) and Statistical Power (β)
> 👍🏼 Rule of thumb: 95% Confidence level and 80% Statistical Power.
I won’t try to give in-depth definitions for confidence level or statistical power.
Both Ronny and Georgi have done a much better job already, so I’m standing on the shoulders of giants.
**A confidence level is a** **measure of the coverage probability** of a [confidence interval](https://www.analytics-toolkit.com/glossary/confidence-interval/). The confidence level represents how often the confidence interval should contain the true Treatment effect. There is a duality between p-values and confidence intervals.
_Full definition from [Analytics Toolkit](https://www.analytics-toolkit.com/) 👉🏼_ **[What does "Confidence Level" mean?](https://www.analytics-toolkit.com/glossary/confidence-level/)**
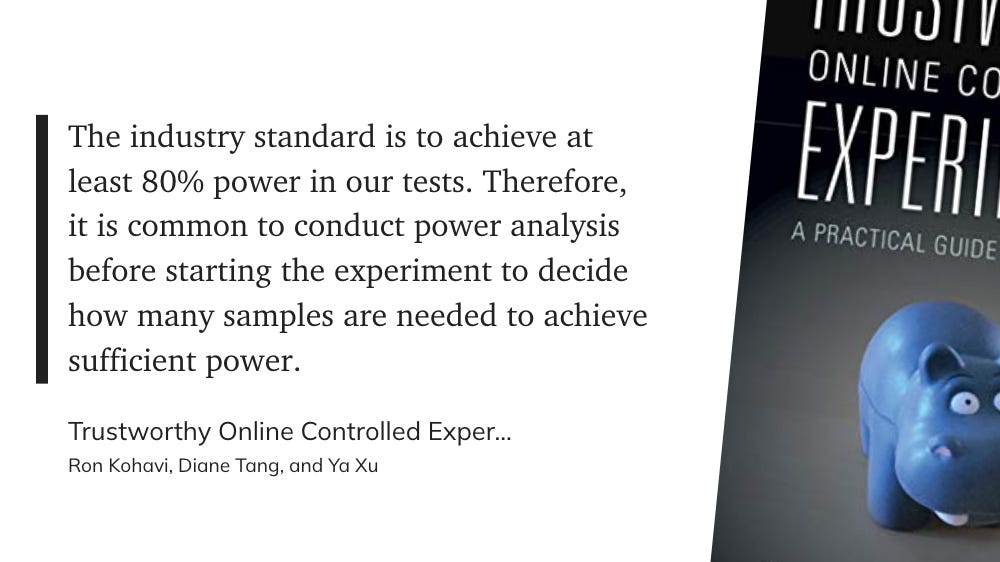
**The statistical power of an [A/B test](https://www.analytics-toolkit.com/glossary/a-b-test/) refers to the test's sensitivity to certain magnitudes of effect sizes.** More precisely, it is the probability of observing a [statistically significant](https://www.analytics-toolkit.com/glossary/statistically-significant/) result at level alpha (α) if a true effect of a certain magnitude is in fact present.
_Full definition from [Analytics Toolkit](https://www.analytics-toolkit.com/) 👉🏼_ **[What does "Statistical Power" mean?](https://www.analytics-toolkit.com/glossary/statistical-power/)**
#### 4. Number of variants to be tested (N)
Georgi summarizes the reason why perfectly on [Analytics Toolkit’s Sample Size calculator page](https://www.analytics-toolkit.com/sample-size-calculator/).
> Tests with more than one variant versus a control need to be analyzed with special methods that account for the [multiple comparisons problem](https://www.analytics-toolkit.com/glossary/multiple-comparisons/) that otherwise arises. The appropriate p-value and confidence interval correction is the [Dunnett's correction](https://www.analytics-toolkit.com/glossary/dunnett%27s-correction/) which means that a sample size calculation should take these corrections into account.
#### 5. Weekly Active “Net” Users (WAnU)
> Your net traffic is not only the users eligible for the test but the users count that historically view or interact with the page template or feature you're trying to improve.
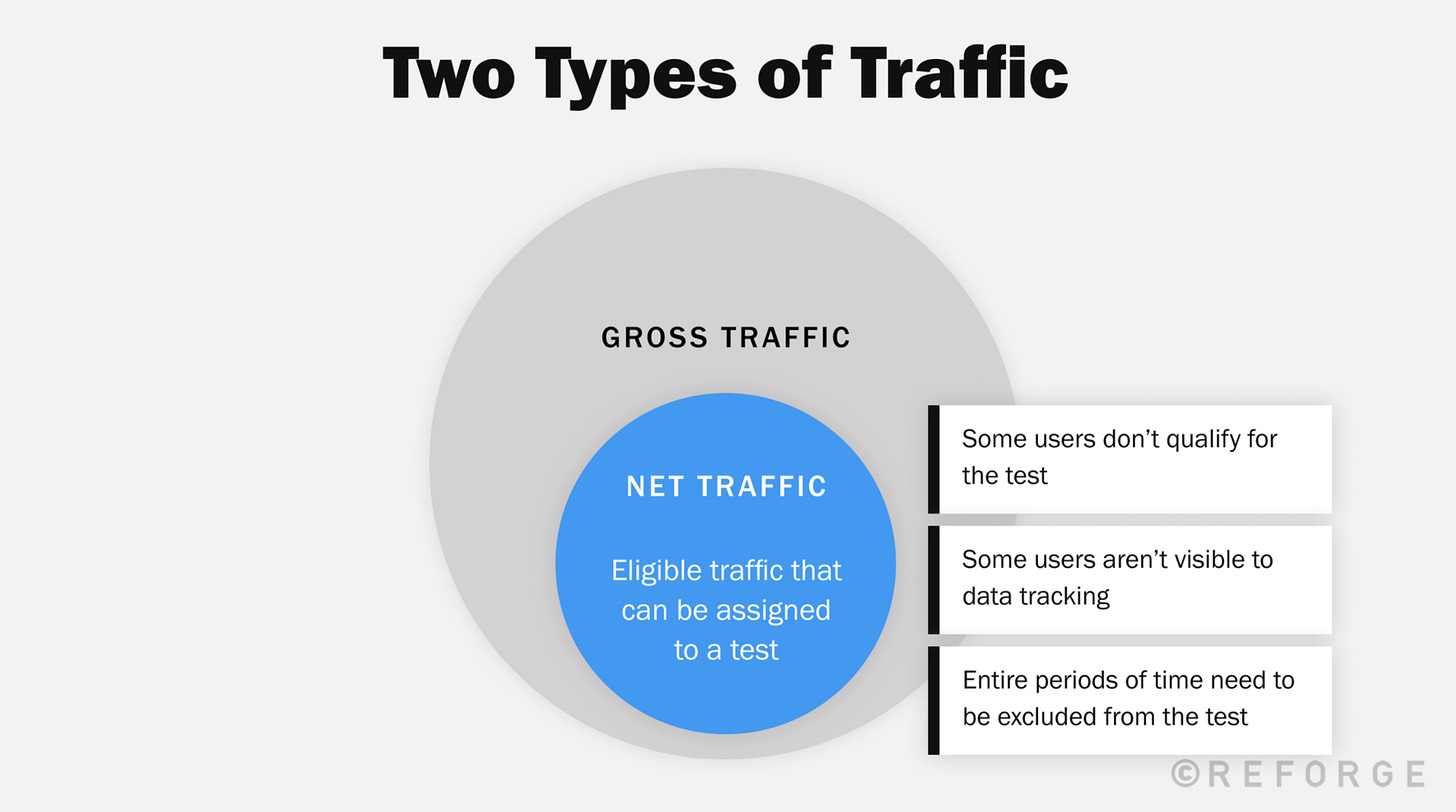
There is no silver bullet answer, every case needs to be assessed individually.
Here are some examples I have on my knowledge base from experience and from Chapter 20, Triggering for Improved Sensitivity, of Trustworthy Controlled Experiments:
- If improving the search page results: Net traffic = total unique users who passed in the search results page over a given period of time.
- If improving the homepage: Net traffic = total unique users who passed in the homepage over a given period of time.
- ⚠️ If improving the CTA on the middle of the homepage (tricky and based on experience): Net traffic = EITHER total unique users who passed in the homepage over a given period of time OR total unique users who passed by the homepage and reached the portion of the page where the CTA is visible.
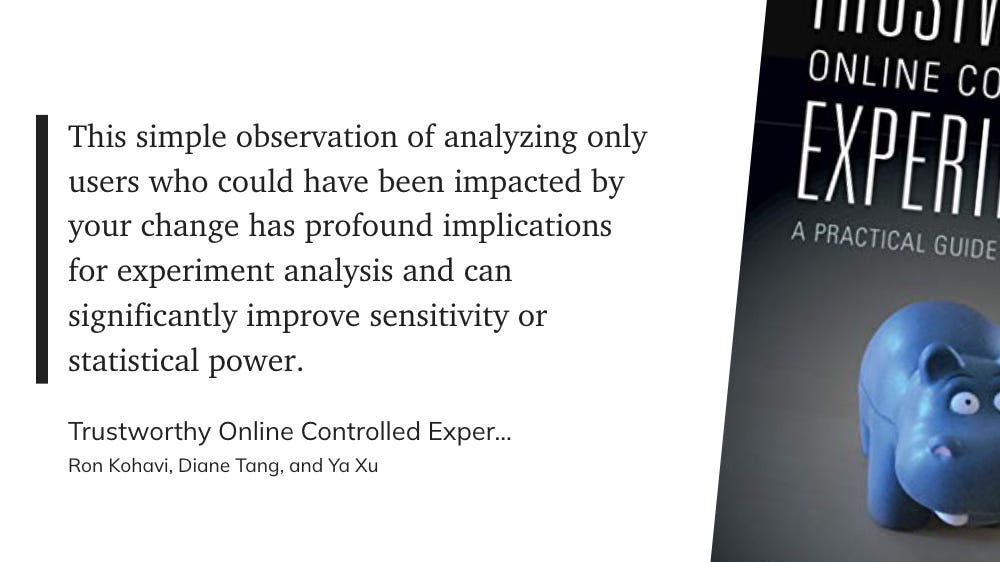
#### 6. Baseline standard deviation (σ)

Standard deviation is a measure of **dispersion** in a numerical data set: how far from the “normal” (average) are the data points of interest.
It can also be said to be a measure of central tendency: the smaller the standard deviation is, the more "clustered" the data is around its center (the [mean](https://www.analytics-toolkit.com/glossary/mean/)).
The larger it is, the more spread the values are.

Google sheets Standard Deviation example
In most cases, you would be calculating STDEV via spreadsheet or via script. In any case, the formula below is what’s being computed under the hood.
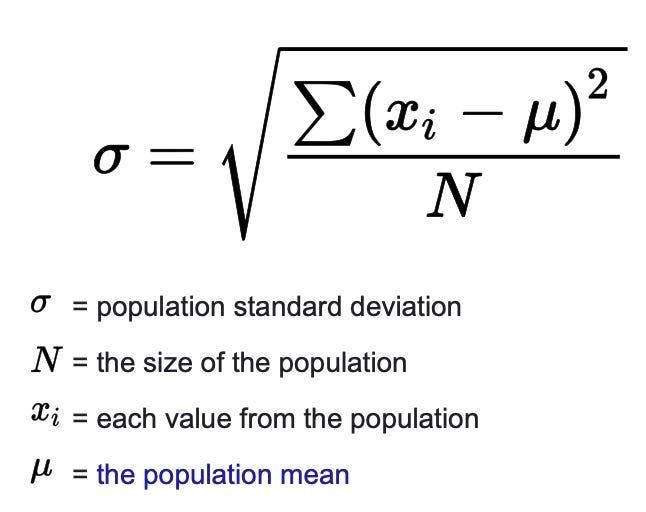
_Full definition from [Analytics Toolkit](https://www.analytics-toolkit.com/) 👉🏼_ **[What is Standard Deviation?](https://www.analytics-toolkit.com/glossary/standard-deviation/)**
## Three methods to calculate sample size for percentage-based metrics
### Our example — eToro landing page
An example will paint a better picture of what we’re doing before crunching numbers.
Imagine we’re part of eToro’s marketing team looking to test multiple splash pages from their Facebook paid ads acquisition growth loop. ([more on growth loops here](https://positivejohn.substack.com/p/january-2022?s=w))
The campaigns are re-targeting audiences, so we’re assuming higher than average intent. The objective of the splash page is to get people to click through the next step.
The click-through rate is high, around 22.8% which we’re using on the calculations below.
[
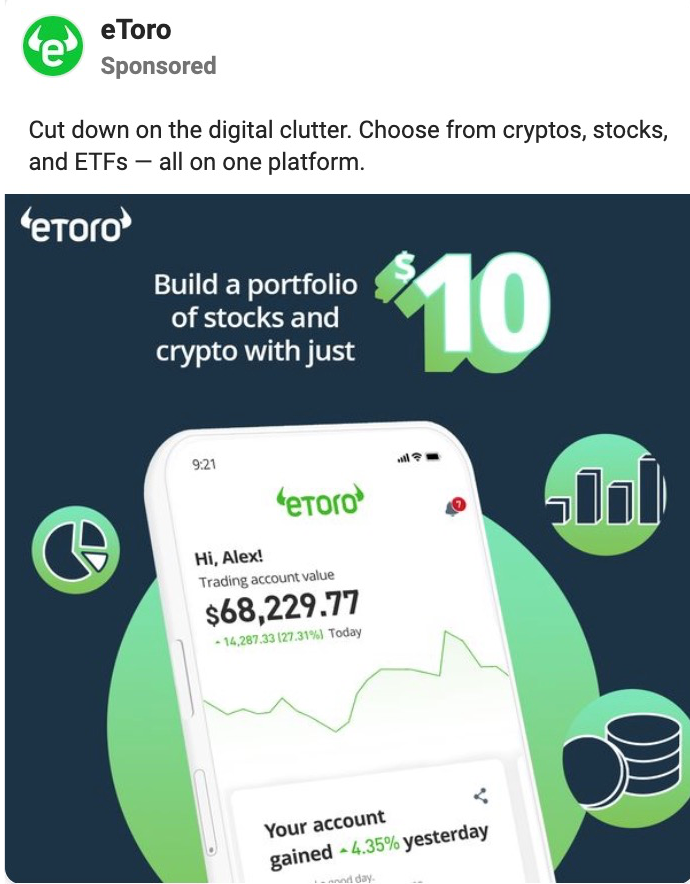
[Facebook ads from Facebook Ad Library](https://www.facebook.com/ads/library/?id=485618316551183)
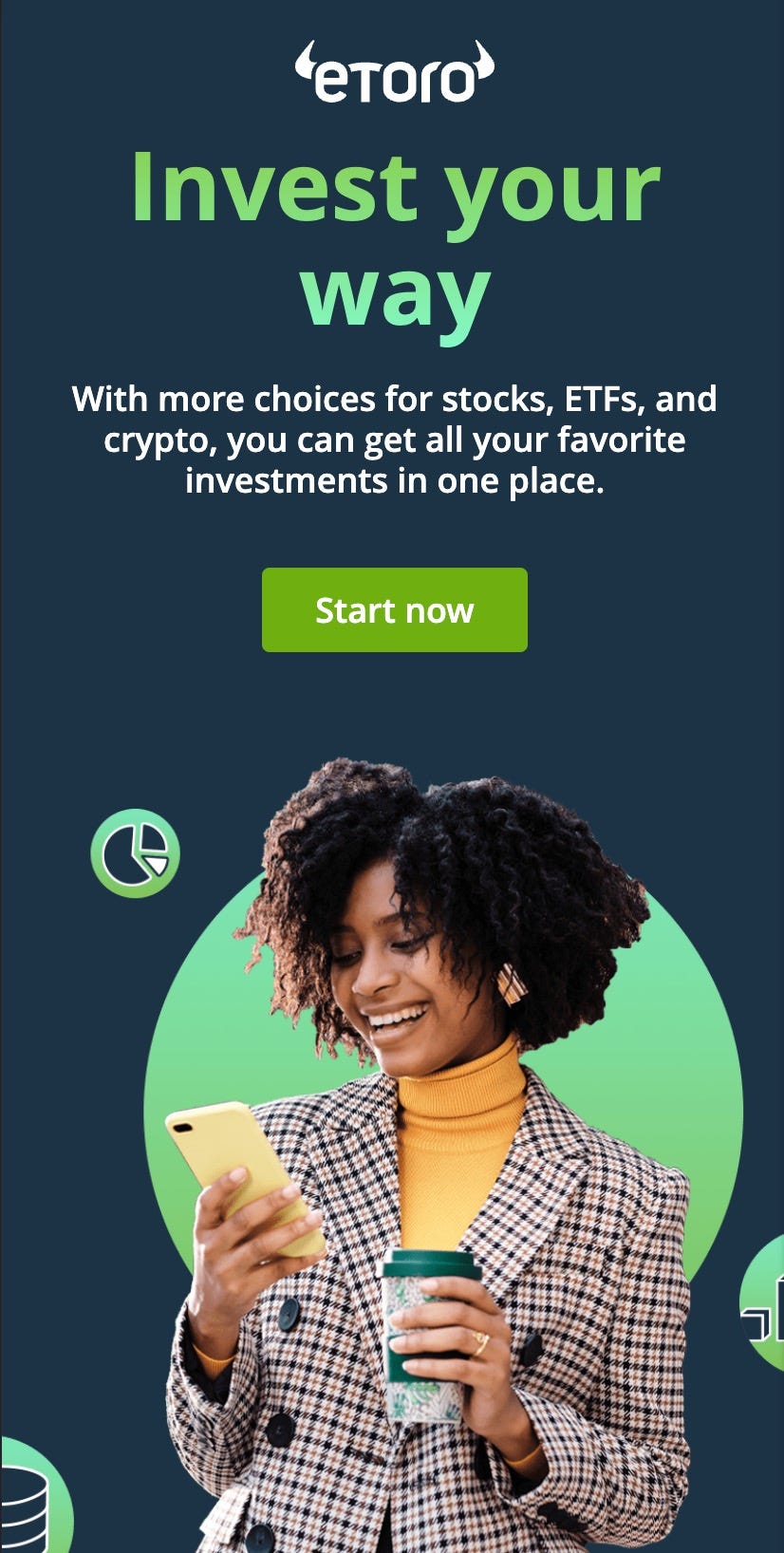
Mobile landing page
### **Using online calculators**
I have two favorites, from CXL and Analytics Toolkit.
#### [CXL calculator](https://cxl.com/ab-test-calculator/) (Free)
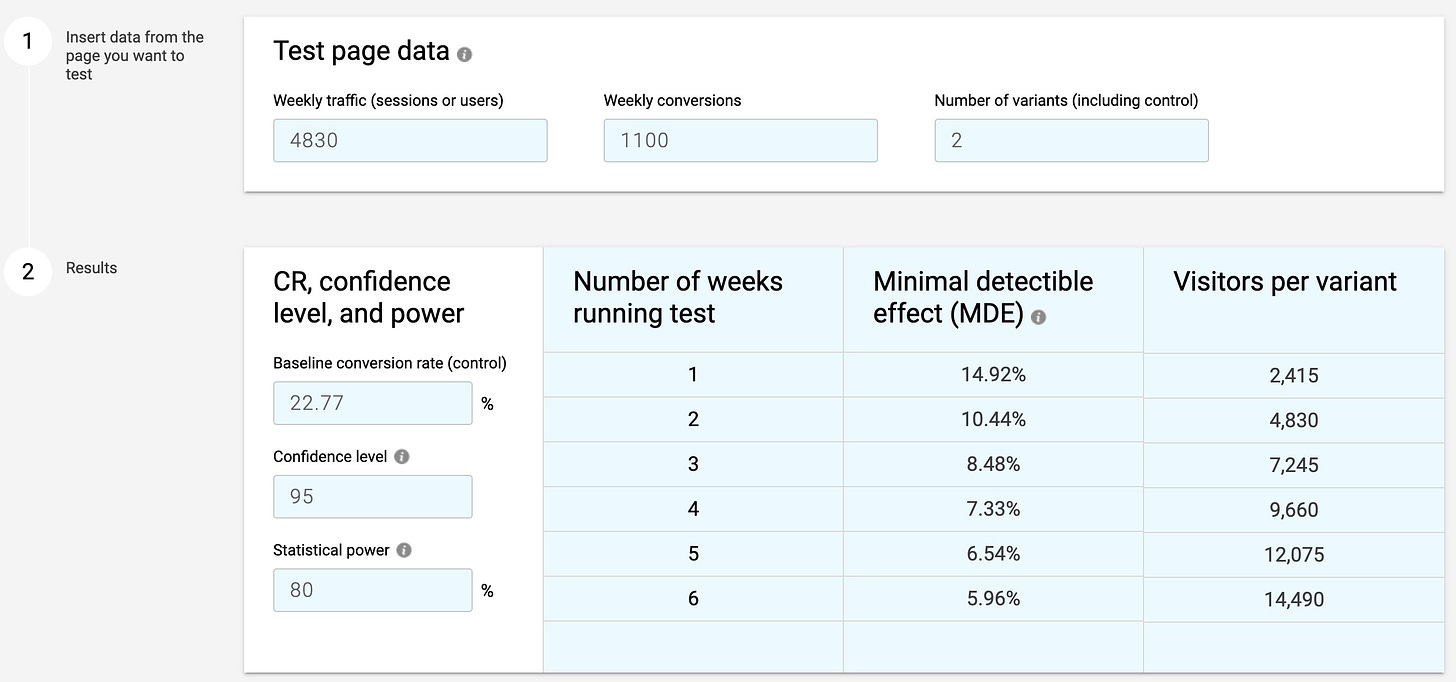
I used this one a lot because it gives you MDE in function of time.
The example above, with its baselines, would help us decide between:
- Running it for 4 weeks with a “difficult MDE” ( >= 7%)
- Going for 6 weeks with a “medium difficulty” (~ 5%)
[Check CXL Calculator](https://cxl.com/ab-test-calculator/)
#### [Analytics Toolkit calculator](https://www.analytics-toolkit.com/sample-size-calculator/) (Starts at $15/month)
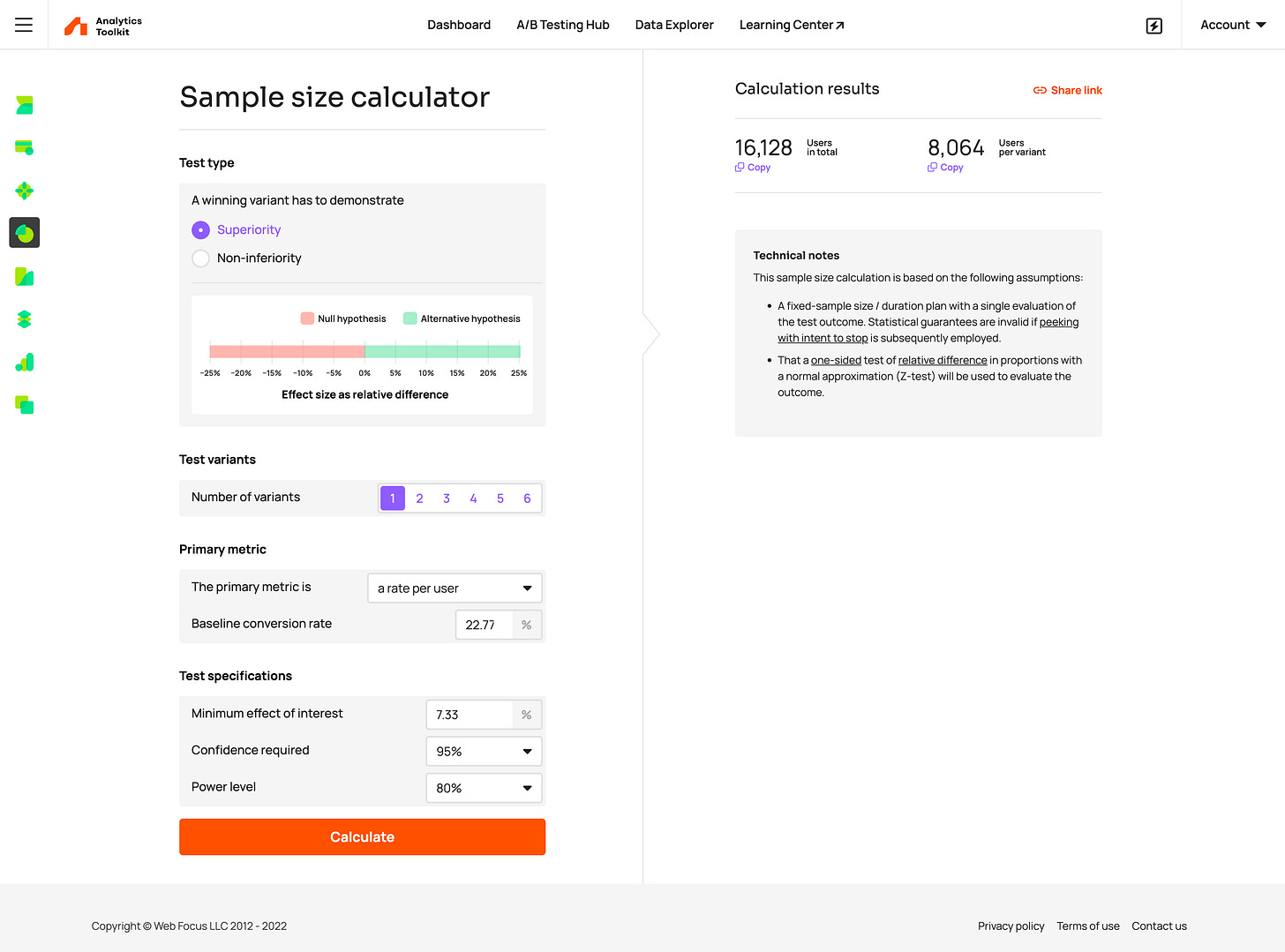
This tool is much superior in multiple ways.
- More control of parameters (e.g. superiority, non-inferiority)
- Documentation and tooltips are extremely user friendly
- I trust the engine.
If Google Analytics or Mixpanel are part of your suite, go for this as they have native integrations.
[Check Analytics Toolkit calculator](https://www.analytics-toolkit.com/sample-size-calculator/)
### **Using spreadsheets**
The single benefit of having these estimations on a spreadsheet is being able to consider multiple page options at the same time for easier prioritization.
I have two favorites, from [Online Dialogue](https://www.linkedin.com/company/onlinedialogue/) and [David Sanchez](https://www.linkedin.com/in/dsanchezdelreal/).
#### Online Dialogue’s calculator
I attribute this one to Online DIalogue because I carry this template since the old school version of CXL’s Conversion mini degree where [Ton Wesseling](https://www.linkedin.com/in/tonwesseling/) would walk you through this model.
- Duplicate the template for each OEC you might be considering to optimize for
- The calculator assumes 80% power
- The tests per year estimation is not awesome and I haven’t re-worked it.
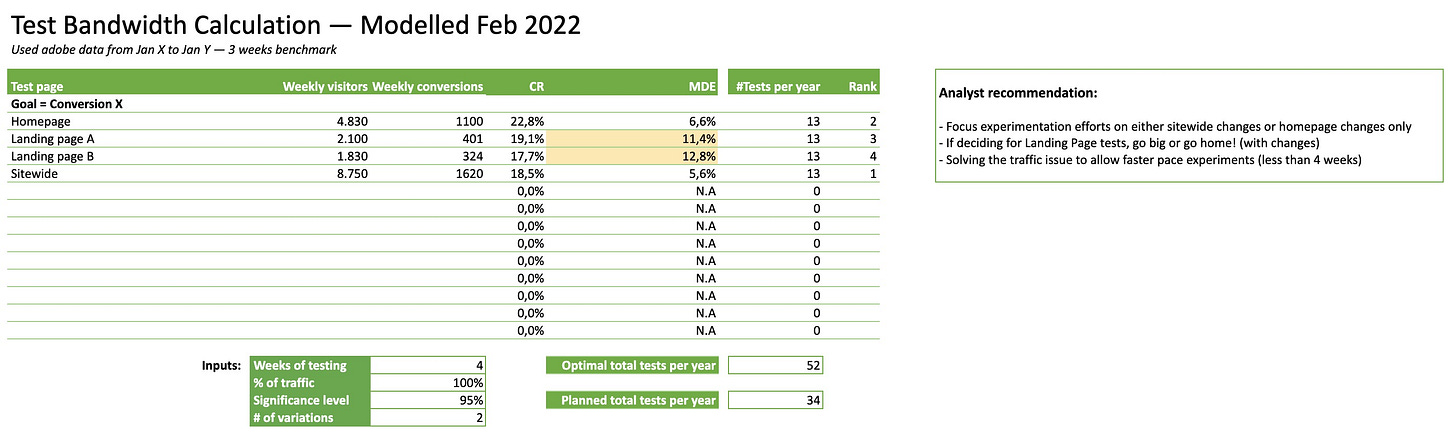
[Copy this template](https://docs.google.com/spreadsheets/d/1wrlQTXv4LTCPbak5feB2hZGb_Nj-EV0jIkx51i96pGA/edit?usp=sharing)
## For continuous metrics
## Two methods to calculate sample size for continuous metrics.
### Our example — Instacart product page (from [Reforge](https://www.reforge.com/experimentation-testing))
Now we’re part of Instacart’s optimization team looking to increase the user average basket size.
We know the average baseline for basket size to be $75 and the standard deviation $43.72
Our test hypothesis implements a recommendation system in the product page in the hopes of reaching a 2% metric lift ($1.50 MDE).
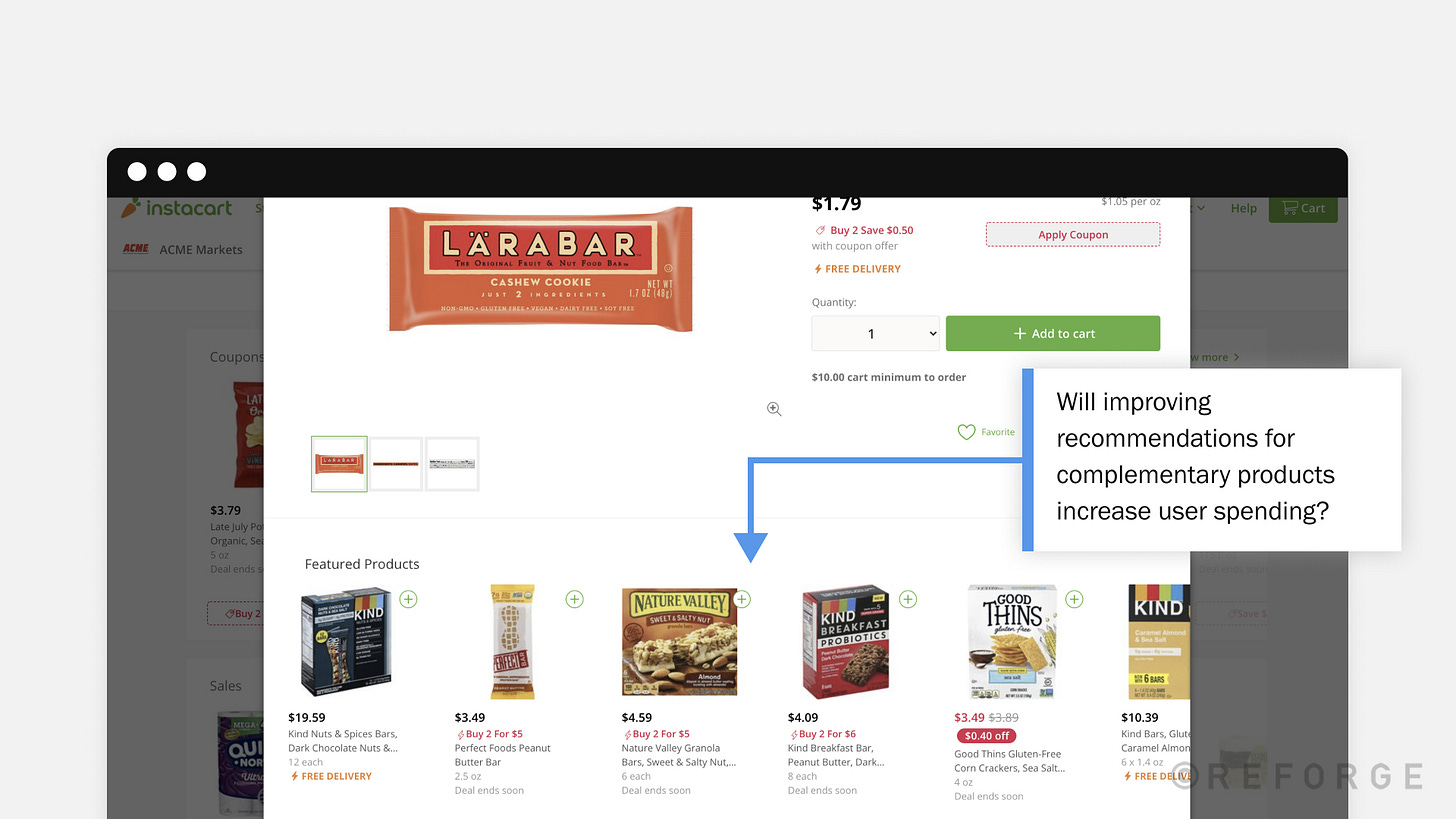
Example from Reforge Experimentation Deep Dive
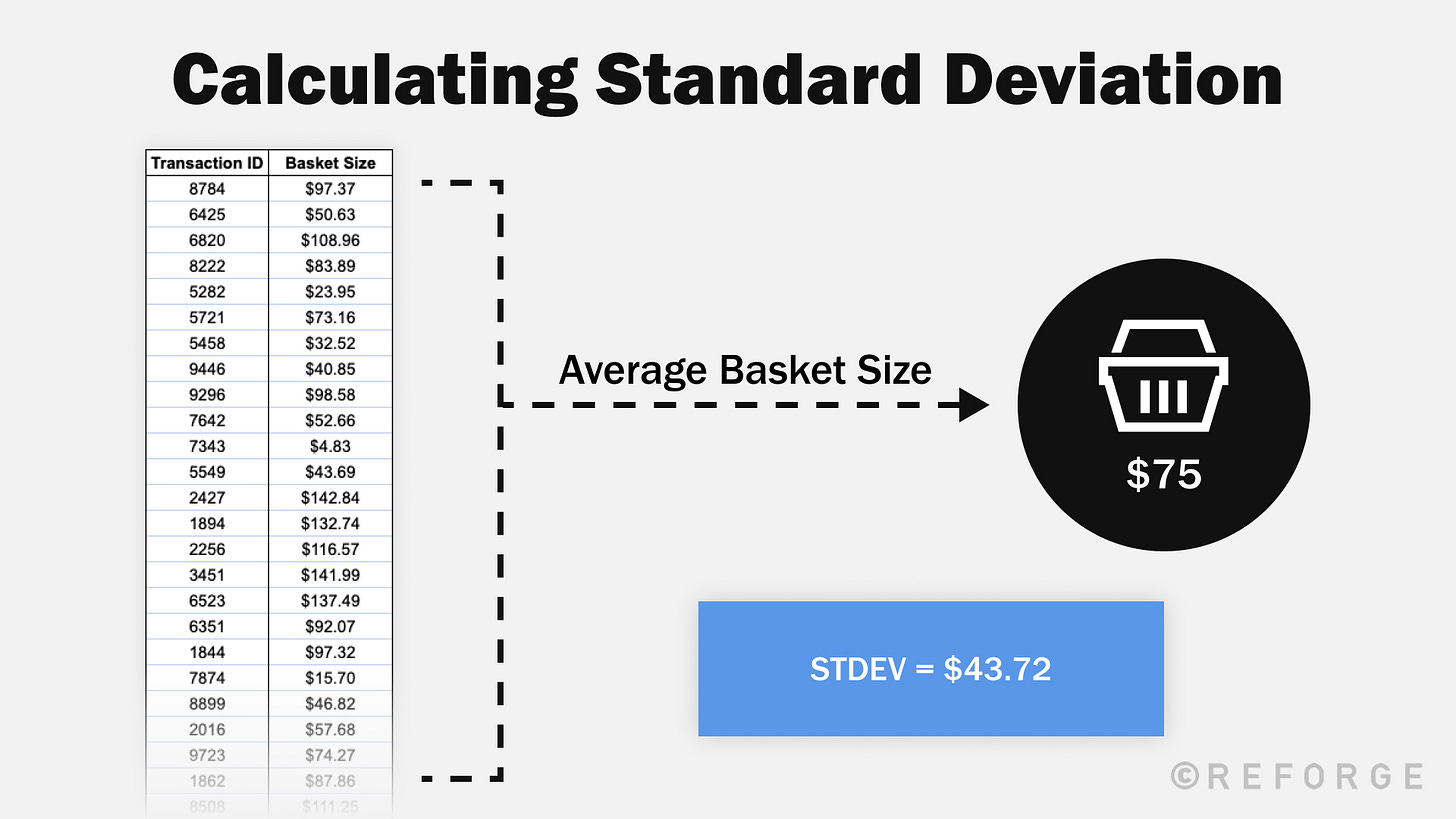
Inputs for calculation.
### **Using online calculators**
#### [Analytics Toolkit calculator](https://www.analytics-toolkit.com/sample-size-calculator/) (Starts at $15/month)
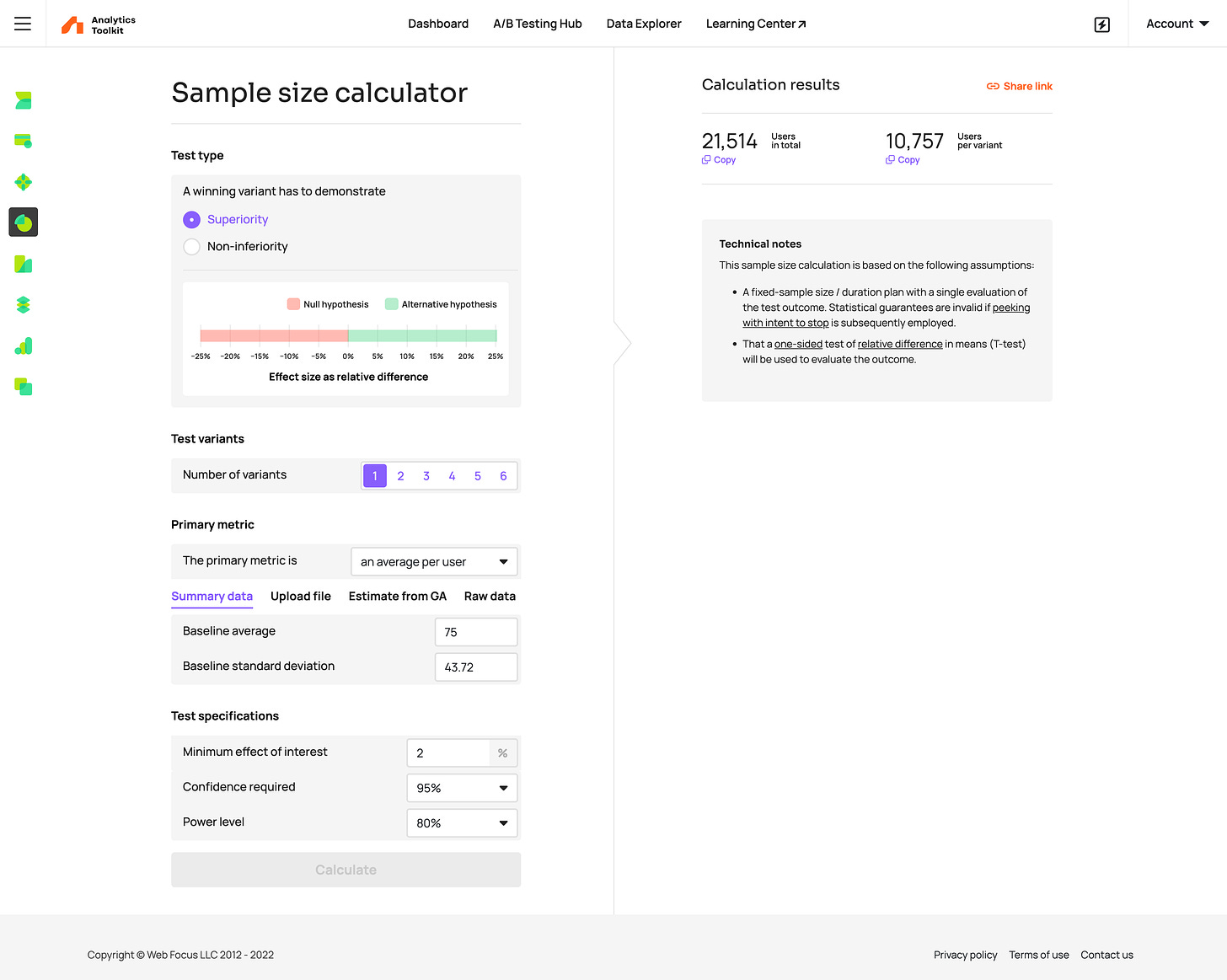
As simple as it gets.
[Check Analytics Toolkit calculator](https://www.analytics-toolkit.com/sample-size-calculator/)
## Test capacity, why does it matter?
From my experience with iTech Media, we understand capacity as a metric that helps us answer “based on the traffic levels we have, how much could we be learning from experiments?”
**I believe reporting on this metric is powerful for communication purposes and to generate top-down accountability for running more experiments**, especially within decentralized organizations where Product is responsible for running experiments.
> Note: This is a program-level metric that is looking to optimise for quantity not quality, and it’s ok. Most experimentation teams started by optimising for quantity (e.g., Booking.com, Microsoft.)
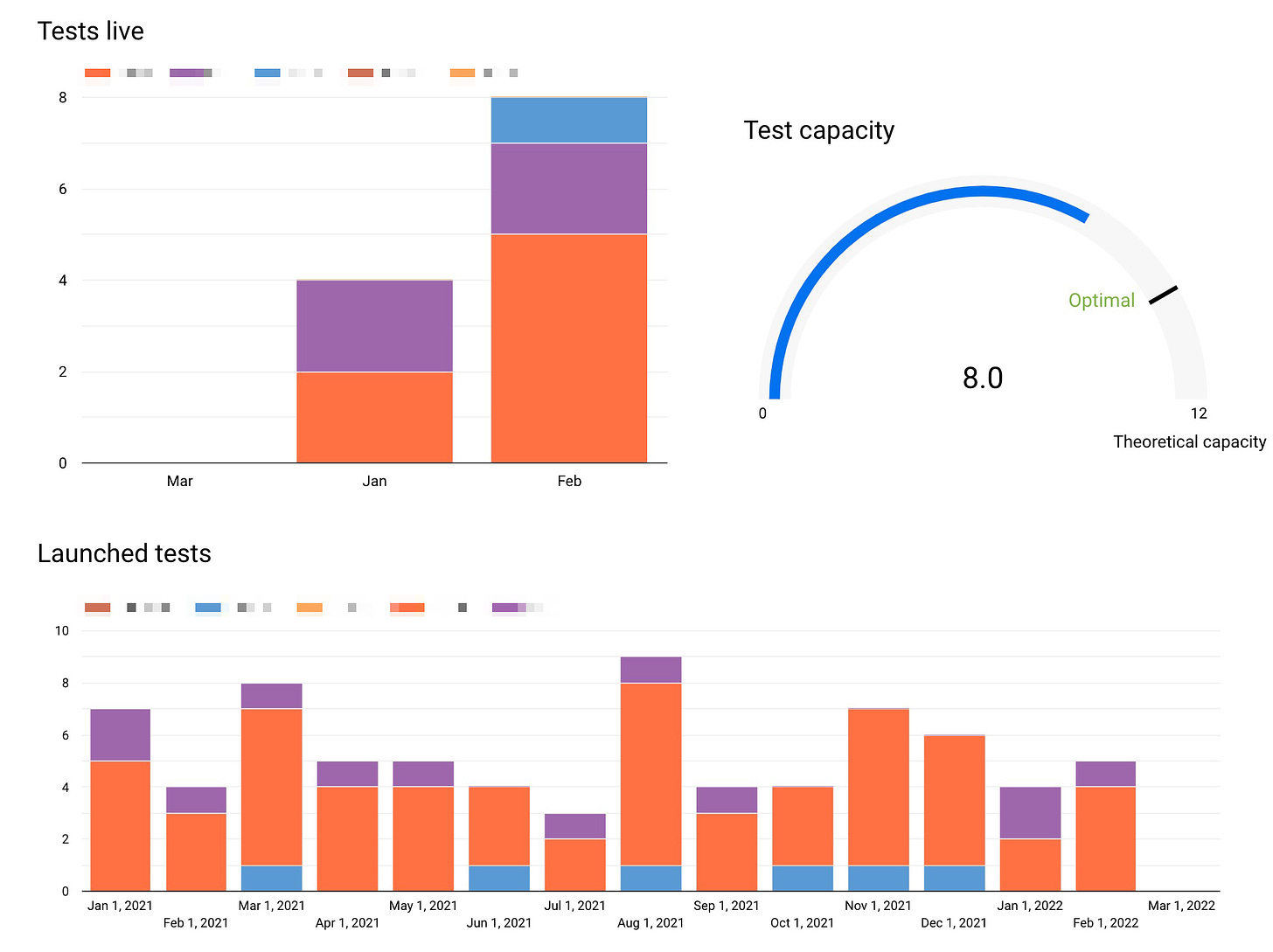
Reporting on test capacity up and down the chain of command
### How to estimate test capacity?
After a lot of discussions about this, I still don’t have a silver bullet answer.
Why? Because it’s highly dependent on how your company operates and how experimentation works on the product.
The technicalities start at _do you test only on stand-alone pages? Could you group pages or templates that have a common feature or component and test them in aggregate?_
The way I do it and recommend others to do is the following:
1. Use spreadsheet calculators to analyze test capacity for your main OEC across multiple pages. Consider the sum of capacity your “theoretical capacity.” Update this metric on a quarterly basis.
2. Monitor both tests launched and tests live by the end of each month.
3. Report on bandwidth usage based on theoretical capacity / tests live by the end of the month.
4. Report and hold accountability for “optimal capacity” based on test live historical data. This is a subjective measurement and it evolves with the intuition of the experimenter.
### [[Frequentist vs Bayesian]]

Bayesian analysis provides much clearer language to explain experimental results.
With the simplistic Bayesian framework I use with my product teams, [read more here](https://www.linkedin.com/pulse/making-product-decisions-bayesian-analysis-john-ostrowski/?published=t), **updating can be seen as an alternative for a sample-size determination that does not require specification of the effect size under the alternative hypothesis.**
[There are white papers that attempt to calculate a sample size for Bayesian testing](https://link.springer.com/article/10.3758/s13428-020-01408-1)
I don’t do it.
# Quote
> Quote
> Quote
---
# Related to